Dynamic Scene Understanding: The Role
of Orientation Features in Space and
Time in Scene Classification
Contributors
- Konstantinos G. Derpanis
- Christoph Feichtenhofer (TU Graz)
- Matthieu Lecce (University of
Pennsylvania)
- Axel Pinz (TU Graz)
- Kostas
Daniilidis (University of
Pennsylvania)
- Richard P. Wildes
Overview
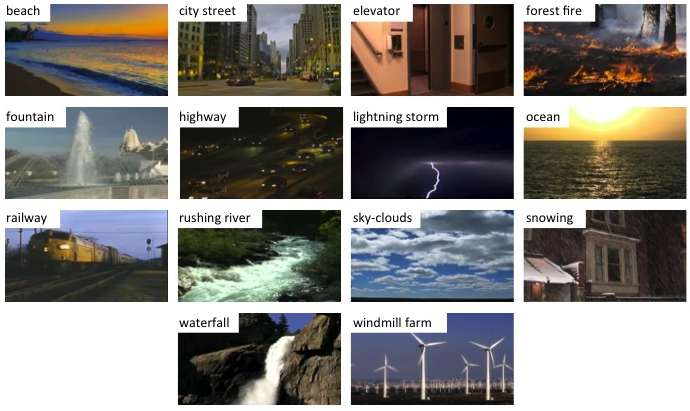
|
Sample
frames of all scenes from the
YUPENN Dynamic Scenes data set.
|
Natural
scene classification is a fundamental
challenge in the goal of automated scene
understanding. By far, the majority
of studies have limited their scope to
scenes from single image stills and
thereby ignore potentially informative
temporal cues. This work is
concerned with determining the degree of
performance gain in considering short
videos for recognizing natural scenes.
Towards this end, the impact of
multi-scale orientation measurements on
scene classification is systematically
investigated, as related to:
- spatial appearance
- temporal dynamics
- joint spatial appearance and dynamics
In addition, a new
data set (YUPENN Dynamic Scenes) is
introduced that contains 420 image
sequences spanning fourteen scene
categories, with temporal scene
information due to objects and surfaces
decoupled from camera-induced ones. This
data set is used to evaluate
classification performance of the various
orientation-related representations, as
well as state-of-the-art alternatives.
Approach
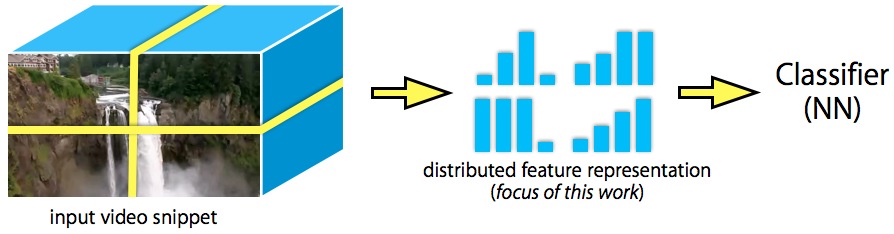
|
Approach
overview. The input
image sequence is spatially
subdivided. Outer scale is
determined by the spatiotemporal
support of the individual subdivided
regions. Relative position of
subdivided regions captures scene
layout. Next, a distributed feature
set is extracted within each
subdivision and concatenated to form
the global feature descriptor, e.g.,
distribution of spacetime
orientation measurements indicating
the relative presence of the chosen
orientation set. Finally, the
feature descriptor is classified
using a nearest neighbor classifier
(NN).
|
There are two key parts to the analysis
of dynamic scene recognition considered in
this work (see Approach overview
above):
1.
a representation based on the
global layout of multi-scale local
feature measurements that are
aggregated across spacetime image
subregions
- spatial appearance: color
and GIST
- temporal dynamics: histogram
of flow (HOF), chaos and
appearance marginalized
spatiotemporal oriented
energies (MSOE)
- joint spatial appearance and
dynamics: chaos fused with
GIST, HOF fused with GIST and
spatiotemporal oriented
energies (SOE); in addition,
colour fused with each of the
spatiotemporal features
|
2. a
match measure between any two
samples under consideration for
classification |
Results
Evaluation
was conducted on two data sets: (i)
Maryland "In-the-Wild and (ii) the new YUPENN Dynamic
Scenes. Since the Maryland
data set contains large camera motions and
scene cuts, it is difficult to understand
whether classification performance of
approaches depends on their success in
capturing underlying scene structure vs.
characteristics induced by the
camera. To shed light on this
question, the YUPENN
dataset is introduced which contains
a wide variety of dynamic scenes captured
from stationary cameras. It is found
that spacetime oriented energies designed
to capture both spatial appearance and
temporal dynamics are the best performer
on the stabilized data set. Overall,
the spacetime oriented energy feature
consistently characterizes dynamic scenes
whether operating in the presence of
strictly scene dynamics (stabilized case)
or when confronted with overlaid,
non-trivial camera motions (in-the-wild
case). The alternative approaches
considered are less capable of such wide
ranging performance.
Supplemental Material
Related Publications
Last updated: Juky 23, 2014 |