Dynamic Texture Recognition Based on Distributions of Spacetime Oriented
Structure
Contributors
- Konstantinos G. Derpanis
- Richard P. Wildes
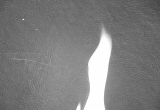 | 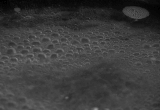 | 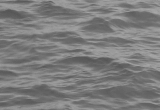 | 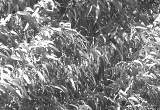 |
Examples of dynamic textures in the real-world. (left-to-right) Fire, boiling water, wavy water, windblown vegetation. |
Overview
A readily observable set of visual phenomena encountered in the natural world are dynamic patterns that are due to the temporal variation (e.g., movement) of a large number of individual elements. Several examples are shown in the figure above. Such patterns, commonly termed dynamic textures, primarily are characterized by the aggregate dynamic properties of elements or local measurements taken over a region of spatiotemporal support, rather than in terms of the dynamics of individual constituents.
Challenge
The goal of the present work is the development of a unified approach to representing and recognizing a diverse set of dynamic textures with robustness to viewpoint and ability to encompass recognition in terms of semantic categories (e.g., recognition of fluttering vegetation without being tied to a specific view of a specific bush). Toward that end, an approach is developed that is based solely on observed dynamics (i.e., excluding purely spatial appearance).
Representation
In our work, local spatiotemporal orientation is of fundamental descriptive power, as it captures the first-order correlation structure of the data irrespective of its origin (i.e., irrespective of the underlying visual phenomena), even while distinguishing a wide range of dynamic patterns of interest. Correspondingly, each dynamic texture will be associated with a distribution (histogram) of measurements that indicates the relative presence of a particular set of 3D orientations in visual spacetime, (x, y, t), as measured by a bank of spatiotemporal filters, and recognition will be performed by matching such distributions.
Analogous to spatial texture discrimination work (e.g., (Knutsson & Granlund, 1983; Bergen & Adelson, 1988; Fogel & Sagi, 1989; Malik & Perona, 1990; Landy & Bergen, 1991)), the texture discrimination model proposed here assumes that two dynamic textures that produce similar spacetime orientation distributions are instances of the same visual category. This approach hinges on the principle that all of the spacetime information necessary for discriminating dynamic texture patterns can be captured by the first-order correlation structure (spacetime orientation) of the data. Although a simplification, this model captures an interesting set of dynamic textures.
Recognition is realized by decomposing a novel input dynamic texture pattern in terms of its constituent spacetime orientations and comparing with a database of samples. The overall approach to dynamic texture recognition is illustrated in the figure below.
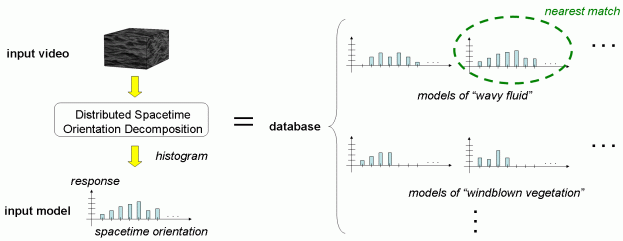 Overview of spacetime texture classification approach. A novel input video is classified by forming its histogram of spacetime oriented energies,
indicative of the relative presence of a given set of spacetime orientations in the
pattern (while being independent of spatial appearance), and followed by nearest
neighbour classification. The class of the input video is defined as the associated
class of the nearest model in the database (in the sense of the chosen similarity
measure, e.g. Bhattacharyya).
Results
The performance of our approach was compared to the popular Linear Dynamic System (LDS) model (e.g., [Saisan, Doretto, Wu and Soatto, CVPR2001, Woolfe and Fitzgibbon, ECCV2006, Chan and Vasconcelos, CVPR2007]) on the standard UCLA database [Saisan, Doretto, Wu and Soatto, CVPR2001]. In addition, controls were introduced to remove the effects of identical viewpoint, as suggested in [Woolfe and Fitzgibbon, ECCV2006]. Our empirical evaluation demonstrates that the proposed approach achieves superior performance over the alternative state-of-the-art methods in the context of shift-invariant recognition.
Further, the usual experimental use of the UCLA database relies on distinctions made on the basis of particular scenes, emphasizing their spatial appearance attributes (e.g., flower-c vs. plant-c vs. plant-s) and the video capture viewpoint (i.e., near, medium and far); for several examples, see supplemental video. This parceling of the database overlooks the fact that there are fundamental similarities between different scenes and views of the same semantic category. In response to these observations, the final reported experiment reorganizes the database into the following seven semantic categories: flames, fountain, smoke, water turbulence, water waves, waterfall and windblown vegetation. Results for this experiment provide strong evidence that the proposed approach is extracting information relevant for delineating dynamic textures along semantically meaningful lines, e.g., over 90% correct classification; moreover, that such distinctions can be made based on dynamic information without inclusion of spatial appearance.
Supplemental Material
Related Publications
- K.G. Derpanis and R.P. Wildes, Dynamic Texture Recognition based on Distributions of Spacetime Oriented Structure, In Proceedings of the IEEE Conference Computer Vision and Pattern Recognition (CVPR), 2010.
- K.G. Derpanis and R.P. Wildes, Spacetime Texture Representation and Recognition Based on a Spatiotemporal Orientation Analysis, IEEE Transactions on Pattern Analysis and Machine Intelligence (PAMI) 34 (6), 1193-1205, 2012.
- R.P. Wildes and J.R. Bergen, Qualitative spatiotemporal analysis using an oriented energy representation, European Conference on Computer Vision (ECCV), pp. 786-784, 2000.
Last updated: February 4, 2013
|